Marcella Cornia, Matteo Stefanini, Lorenzo Baraldi, Rita Cucchiara
Proceedings of the IEEE/CVF Conference on Computer Vision and Pattern Recognition (CVPR 2020)
June 2020
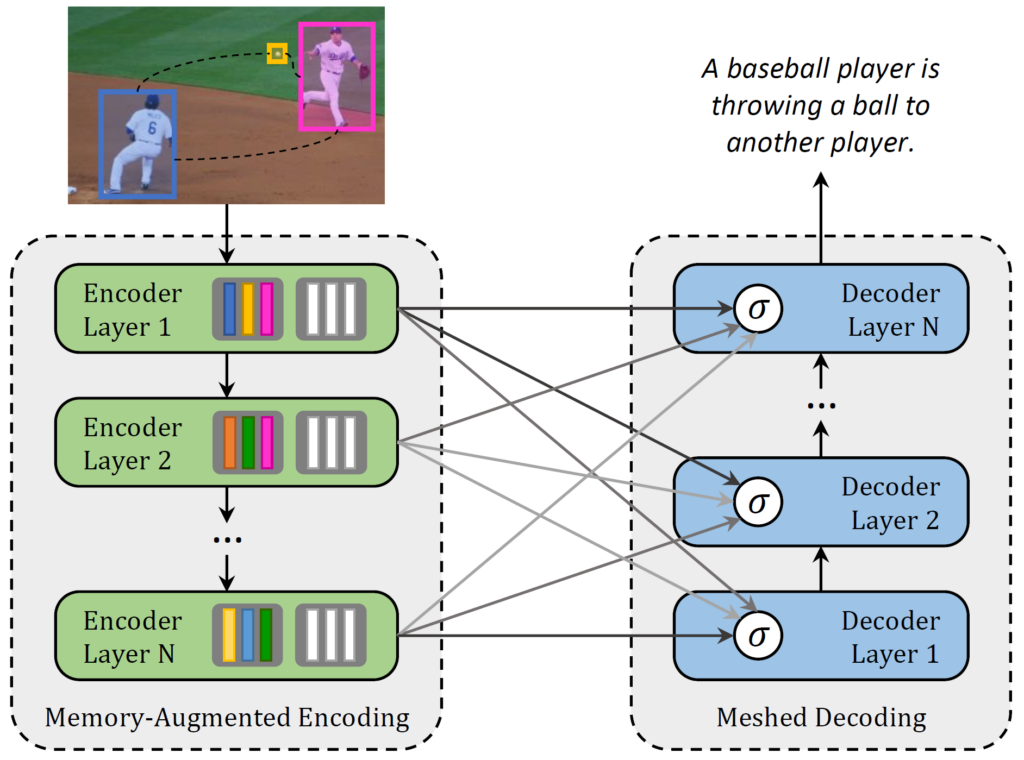
Abstract
Transformer-based architectures represent the state of the art in sequence modeling tasks like machine translation and language understanding. Their applicability to multi-modal contexts like image captioning, however, is still largely under-explored. With the aim of filling this gap, we present a Meshed Transformer with Memory for Image Captioning. The architecture improves both the image encoding and the language generation steps: it learns a multi-level representation of the relationships between image regions integrating learned a priori knowledge, and uses a mesh-like connectivity at decoding stage to exploit low-and high-level features. Experimentally, we investigate the performance of the M Transformer and different fully-attentive models in comparison with recurrent ones. When tested on COCO, our proposal achieves a new state of the art in single-model and ensemble configurations on the Karpathy test split and on the online test server. We also assess its performances when describing objects unseen in the training set.
Type: Conference Paper
Publication: International Conference on Pattern Recognition (ICPR 2022)
Full Paper: link pdf
Code: link github
Please cite with the following BibTeX:
@article{cornia2019m,
title={M $\^{} 2$: Meshed-Memory Transformer for Image Captioning},
author={Cornia, Marcella and Stefanini, Matteo and Baraldi, Lorenzo and Cucchiara, Rita},
journal={arXiv preprint arXiv:1912.08226},
year={2019}
}